Contextual learning allows AI agents to adapt to environmental changes using four key components: State, Transition, Reward, and Action. Previously, AI agents successfully handled the first three components, but adaptation based on actions remained problematic and required retraining when adding new actions.
The Headless-AD model, based on the Distillation algorithm, solves this problem with three main changes: elimination of the finite linear layer, coding of actions with random vectors, and introduction of context. This allows the model to automatically adapt to new actions without sacrificing quality.
In experiments, Headless-AD showed a significant advantage. In recommendation system tasks such as Contextual Bandits, the model was able to recommend 5 times more products than a trained AD model without having to retrain for a new set of products. Also in the “Multi-Armed Bandits” task, Headless-AD showed results at a custom algorithm level.
Source: Ferra
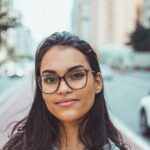
I am a professional journalist and content creator with extensive experience writing for news websites. I currently work as an author at Gadget Onus, where I specialize in covering hot news topics. My written pieces have been published on some of the biggest media outlets around the world, including The Guardian and BBC News.