All current generative AIs have the potential to hallucinate, which is one of the fundamental problems with technology. In order to minimize the problem, Google Created DataGemma, an AI that can fix itself.
Announced this Thursday (12) The tool consists of models trained exclusively on data available on Data CommonsOpen database maintained by Google. However, unlike the content circulating on the internet, the platform’s information is located on the websites of well-known institutions.
THE DataGemma acts on Google model responses in two ways: RIG (Retrieval-Intermittent Manufacturing) and RAG (Retrieval-Augmented Manufacturing).
In the first method, RIG, DataGemma creates a “draft” of the response and then compares the result with content available on Data Commonsfixes everything that can be adjusted with the general database.
In RAG it’s the other way around: first, The model checks if the answer to the user’s question is available in Data Commonsfrom this data an answer is generated. According to Google, this alternative reduces the likelihood of hallucinations.
“Our goal is to use Data Commons to improve the logic of LLMs (large language models) by supporting them with real-world statistical data that can be traced back to their origins,” said Prem Ramaswami, director of Data Commons at Google. The result, he said, is “more reliable” AI.
Currently, DataGemma is only available to researchers, with the possibility of expanding access in the future. If all goes well, the solution could be crucial to implementing generative models in Google’s search engine.
The solution also has its flaws
Clearly DataGemma is not perfect. THE The first problem with this solution is the Data Commons data limitation — If the information is not available on the platform, it is not possible for the model to verify its accuracy. Unfortunately, this happens in many scenarios.
The tool can check the consistency of scientific data, such as economic data for a particular country, but I can’t guarantee the release date of Taylor Swift’s latest hit is accurate.
According to Google, DataGemma failed to extract relevant information 75% of the time from experimental cases. Moreover, although the content was present in the database, the model was not able to access this content to formulate the correct answer.
Moreover, DataGemma also makes mistakesThe researchers found that when using the RAG method, the model gave incorrect answers on 6% to 20% of trials, while RIG was more efficient, allowing the AI to extract data 58% of the time.
THE DataGemma principle is the same as commercial generative models: The larger the database available, the more accurate the AI becomes. Therefore, with increased training and improvements to the model, the tool tends to become more accurate and effective in correcting the responses.
In any case, the solution is still far from perfect and does not solve the problem of hallucinations from generative AIs, which once again shows that: Chatbots are not always 100% accurate.
Source: Tec Mundo
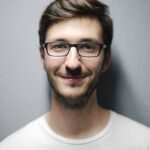
I am a passionate and hardworking journalist with an eye for detail. I specialize in the field of news reporting, and have been writing for Gadget Onus, a renowned online news site, since 2019. As the author of their Hot News section, I’m proud to be at the forefront of today’s headlines and current affairs.