Rafael Ataide’s photo.
You can’t make decisions without data. This is a fact that most companies accept today, with the inclusion of new technologies that we have experienced over the years. This is great news because it means data is being collected and used extensively. But at the same time, another problem arose: the low quality of this information.
According to a study from IBM, poor data quality causes an average financial impact of $9.7 million per year. This is a growing concern in the business world with the development of artificial intelligence. F5’s State of AI Implementation Strategy report shows that 72% of organizations using AI face challenges with data quality and scalability.
The saying “garbage in, garbage out” or “garbage in, garbage out” explains the situation very well. When we make decisions on the wrong basis, the result will naturally be trouble. It’s like cooking with spoiled ingredients: it doesn’t matter whether the recipe is correct or not, the food will still have to be thrown away.
So what is low quality data in practice? We can talk about a number of factors. They may be incomplete, outdated, inaccurate, irrelevant, disorganized, corrupt, partial, inconsistent, ambiguous, misleading, manipulated, or even outright wrong.
It is necessary to be aware of all these possibilities in order for the information collected to be truly useful and useful. The risks are not just financial; compromised data can influence internal, operational, marketing and many other decisions and harm, for example, the company’s image or the coexistence of the team.
In order to be protected from these dangers, a quality standard must be established. Generally, this refers to an organizational policy that all employees and leaders must follow. But this can’t just happen on paper! It is necessary to ensure that the applications are compatible, which can be done through training or with the help of a company that specializes in dealing with data and extracting the best from it.
This data management will be reflected in daily life, the use of appropriate programs and the performance of the team. Here are some examples of what should be included in the policy:
- Data cleaning: Ensure removal of incorrect data;
- Verification and verification: always check the source and quality of data;
- Continuous update: Always keep data up to date;
- Appropriate tools: invest in tools that guarantee data integrity;
- Transparency: Being open about the information collected.
These steps can now take a company to a new level of quality that the business world will welcome. Remember: It’s not about believing any data that appears in a spreadsheet, it’s about making sure it’s the right basis for your choices.
*Having experience in artificial intelligence and neuromarketing, Rafael Ataide has already managed e-commerce, CRM strategies and implemented machine learning algorithms in multinational companies. Today, he leads Adtail’s Data and Technology Center as director, driving innovation and the use of artificial intelligence to streamline day-to-day operations and customers.
****
*Having experience in data science and artificial intelligence, Rafael Ataide has already managed e-commerce, CRM strategies and implemented machine learning algorithms in multinational companies. Today, he leads Adtail’s Data and Technology Center as director, driving continuous innovation and processes to streamline day-to-day operations and the customer.
Source: Tec Mundo
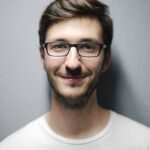
I am a passionate and hardworking journalist with an eye for detail. I specialize in the field of news reporting, and have been writing for Gadget Onus, a renowned online news site, since 2019. As the author of their Hot News section, I’m proud to be at the forefront of today’s headlines and current affairs.