artificial intelligence (AI) It is increasingly present in our lives. At the moment, there are few people of a certain age who have not asked questions on any topic. ChatGPT. There was even a woman who, thanks to one of these consultations, received the long-awaited diagnosis for her son. And disease diagnosis is one of the areas in which AI is developing the most. However, it is important to be careful; because, for example, poor preparation can lead to racial or gender bias.
This was reported by a group of scientists from Imperial College Londonwith a study published in Radiology: artificial intelligence. In it you see the results of a study aimed at comparing results chest x-ray analyzed basic AI model or the one that the researchers themselves refer to.
Thus they saw that important prejudices were being made. Both are associated with patients’ gender and ethnicity. It is logical that attention should be paid to this in order to avoid incorrect diagnoses. And while AI is designed to make our lives easier, it doesn’t seem ready to run unsupervised just yet. Especially at the stage education.
What is the difference between the base model and the reference model?
Artificial intelligence algorithms are usually trained using known data in which general parameters are searched. For example, AI designed to analyze mammograms is trained on thousands of photographs of mammograms, healthy or with tumors, so that the program finds common factors associated with different diagnoses and is able to distinguish between them as new data becomes available.
But such training can be carried out in two different ways. On the one hand, there are basic models, which contains a huge amount of unlabeled data. That is, the AI is not told where they came from, but is given the opportunity to find its origin. similarities and differences.
On the other hand, we have reference models. They are much more specific because the data that is input to train the AI is labeled. For example, in the case of a mammogram, information such as the presence of abnormalities in the breast will be indicated, as well as, for example, the age or ethnicity of the patient. The more labels the better.
Racial and gender bias in AI
The authors of this study wanted to see if AI using basic models could make an accurate diagnosis. To do this, they took an algorithm designed to study chest x-ray.
The one taken initially was trained on a basic, unlabeled model. But on the other hand, the researchers built another model trained using 127,118 perfectly labeled x-rays.
Once this was done, it was decided to use an AI algorithm to study the chest X-rays of 42,884 patients. men and women, white, black or Asian.
The AI diagnoses were tested by trained radiologists, who found the labeled model to perform significantly better. For white men there was not much difference, but for women, blacks and Asians they found greater bias. First of all, they saw that the “no discovery” label dropped. from 6.8% to 7.8% for womenand fluid accumulation in the lungs decreased from 10.7% to 11.6% for black patients.
Why is this and what can we do?
Traditionally, clinical trials and research have been conducted primarily with volunteers. white men. More recently, we began to look for ways to solve a problem that could have very serious consequences.
For example, if a clinical trial is conducted only in men, the appropriate dosage for women may be incorrectly calculated, causing them to have significantly more side effects. And unless research studies include black people, knowledge about how certain diseases, for example, affect them may be very limited.
All this has led to the fact that the data used to train AI algorithms also comes primarily from white men. If they are entered directly, without supervision, as in the basic model, we may not realize it, and artificial intelligence introduces very large errors, which then will change the results.
For this reason, the authors of this study advise to always review the data on which the AI was trained before you start using it. This should be applicable to any field, but it is especially sensitive to medical issues, as they saw in their research. It will take some time, but the diagnosis can be much more accurate. After all, this is exactly what is achieved when introducing new technologies in the field of medicine.
Source: Hiper Textual
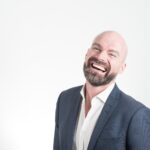