Nvidia released the Hopper architecture in March in the form of the H100 accelerator for data centers. Now the manufacturer has published a 71-page whitepaper detailing the expected performance and build of the massive monolithic GPU. There are two versions as pluggable card and server module, both using GH100 GPU larger than 800mm.2nd† Both have 80GB memory, 50MB L2 cache, PCIe 5.0 and fourth generation NVLink.
The pcie version has 114 of 144 streaming multiprocessors enabled for 14,592 cuda cores and 456 tensor cores. With five hbm2e stacks, 10 of the 12 controllers are used, each with a 512-bit bus width. TDP is 350 watts, a feature that doubles the sxm5 module. Enabled 132 SMS for 16,896 cuda cores and 528 Tensor cores, also used 5 stacks of hbm3. If performance is not specified per version, it refers to the sxm5 version, which can be significantly faster due to the higher core count and doubled tdp.
FP32 performance triples
Nvidia has taken important steps in production thanks to TSMC’s N4 process. Transistor density is 50 percent higher compared to Ampere. As a result, the number of fp32 shaders in each stream multiprocessor can be doubled. Along with a promised improvement in clock speed, performance should be three times higher than the previous generation.
The fp8 number format is still so new that IEEE’s standard implementation has been taken directly. This now calculates the format twice as fast as when it was rendered as fp16. There are two formats to choose from for floating point calculations. E4M3 offers greater accuracy where E5M2 allows greater numbers. The number of the result of the matrix operation can even be given in four forms.
The strong can also be wise
Nvidia wants to use Hopper’s computing power as efficiently as possible. Therefore, dynamic programming instructions have been added. For problems where previously calculated results are used repeatedly, they can be retained more effectively. For example, in optimization problems, the solution can be speeded up 7 times in the best case, making repetitive computations unnecessary.
Where previously the task distribution unit was a 128 shader sm, this has now been expanded. Multiple SMS can be deployed together in a cluster and access the cache of other processors. This should be much faster than reloading the data. For some applications, this can result in a doubling of performance.
Faster Tensor Cores for Matrices
Tensor kernels are used for a specific computational task. When multiplying two matrices, a third matrix has to be calculated for the result, and that’s all these kernels can be used for. The application of matrices in the training of neural networks is so important that it is given its own set of computing cores.
Fourth generation Tensor cores double the performance per clock for all supported number formats. Since kp8 was previously rendered as kp16, even a factor of 4 is achieved there. Performance is increased by another 50 percent due to more cores with higher clock frequency. Fast memory is useful for transferring matrices to Tensor cores. Tensor Memory Accelerator is now responsible for this data transfer to free up sm, resulting in higher performance.
The new Transformer engine uses an algorithm to train an AI for speech recognition. Performance gains can be achieved by adjusting the accuracy at each step between fp8 and fp16. Therefore, the previous result is used to estimate the required precision in the next calculation. The fp8’s lower accuracy and smaller number space and desired result are taken into account.
Video decoders, but not for picture
The video decoders built into this server GPU are not used to generate an image output. Video material must be decoded for training deep learning models. The number of simultaneous streams that can be processed doubled to 340 full HD streams. It has support for H.265, H.264 and VP9. In addition, up to 6,350 Full HD images per second can be compressed into a jpeg file.
Focus on AI
All these numbers show that Nvidia has put as much AI performance as possible in a massive chip with this generation. In the field of classical vector computing, AMD sometimes stays ahead of the green camp with MI200 and CDNA2. Remarkably, the opponent is already using chiplets or tiles. Nvidia has not used this approach for now, so there may be successes that can be touched upon in the future.
Source: ComputerBase
Source: Hardware Info
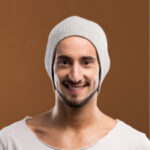