By Júlio Eduardo Martins.
The world of technology is constantly evolving, shaping our society and lifestyle. Two prominent trends in the last decade are Edge Computing and Machine Learning.. Edge Computing refers to the centralization of data processing, while Machine Learning is an artificial intelligence discipline that allows systems to learn and make decisions based on data.
In this article, we will examine how these two technologies can come together to support the development of innovative solutions, the challenges facing this integration, and what the promising future of this union is.
What is Edge Computing and Machine Learning?
As discussed in previous articles, Edge Computing – or Edge Computing – means a Computational approach that transfers some of the data processing from the cloud to devices or locations close to the source of origin. This strategic change aims to reduce latency and increase the efficiency of real-time applications.
This means that with Edge Computing, devices can make decisions quickly, making it ideal for IoT applications, autonomous vehicle operation and other real-time applications.
Machine Learning has revolutionized the ability of systems and devices to learn and adapt based on data. This technology is present in our daily lives today, from voice assistants to online product recommendations. The ability to analyze large volumes of data and derive valuable insights makes Machine Learning an incredibly powerful tool.
The combination of these two technologies (Edge Computing and Machine Learning) creates a perfect marriage. Ultimately, devices at the edge can leverage Machine Learning models to make autonomous decisions by adapting to conditions in real time. For example, a driverless car that processes data locally eliminates unforeseen obstacles based on Machine Learning training.
Despite significant advances in these two technologies in recent years, this union still faces significant obstacles. Edge devices often have limited resources compared to cloud servers. Running complex Machine Learning algorithms on resource-limited devices can be quite challenging.
Another important issue is ensuring data security. By decentralizing processing, Edge devices may be more vulnerable to physical attacks and security management of Machine Learning models are vital in this scenario.
Despite the challenges, these points will tend to be overcome as technology advances, and the integration between Edge Computing and Machine Learning will continue to play an increasing role as a leading actor in new solutions. We can expect advances in smart cities, healthcare, manufacturing and transportation that will transform many of the environments we interact with on a daily basis.
The union between Edge Computing and Machine Learning will surely revolutionize the way we live with technology and the world around us. Although there are various challenges ahead, the potential for innovation and improvement in various sectors is exciting as it promises a future full of opportunities and benefits for humanity.
As these two technologies evolve, we must prepare to enjoy this upcoming digital transformation.
****
*Júlio Martins is Director of Innovation at Roost, a technology company specializing in Edge Computing solutions.
Source: Tec Mundo
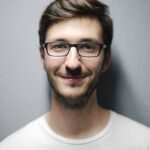
I am a passionate and hardworking journalist with an eye for detail. I specialize in the field of news reporting, and have been writing for Gadget Onus, a renowned online news site, since 2019. As the author of their Hot News section, I’m proud to be at the forefront of today’s headlines and current affairs.