When we use AI, we rarely think about how it “learns.” In fact, the learning process of neural networks is similar to ours: they learn to predict events and recognize objects by examining large amounts of data through trial and error. However, developers are faced with the question of where to get so many examples, and this is where synthetic data comes to the rescue. Experts emphasize that economic reasons play an important role in their creation, since modeling real situations can be very expensive and time-consuming.
Synthetic data is readily available and relatively inexpensive. For example, if you need to train a neural network to detect large-sized items on a conveyor belt, you can create many virtual “stones” for this purpose. As Yuri Chainikov explains, with the help of 3D modeling, you can have millions of options to help train a neural network. Generating such data takes only a few seconds on a regular computer, and the result is the amount of information needed for training.
Despite its similarity to real data, synthetic data also has its limitations. To build effective neural networks, the data needs to look real. For example, if you’re training a medical neural network, it’s important to use dialogue that’s choppy and natural-sounding, similar to real conversations. Synthetic data helps create many variations of rare situations, which allows the neural network to better understand the problem and develop generalization capabilities. This approach, known as the Monte Carlo method, is used to generate random data within certain limits, making learning more diverse and efficient.
Source: Ferra
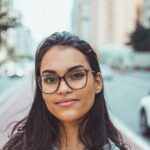
I am a professional journalist and content creator with extensive experience writing for news websites. I currently work as an author at Gadget Onus, where I specialize in covering hot news topics. My written pieces have been published on some of the biggest media outlets around the world, including The Guardian and BBC News.