There are various definitions and a broad discussion about the concept of Artificial Intelligence (AI). In general, technology can be defined as: the ability of a system or mechanism to simulate human intelligence🇧🇷
IBM, for example, has a great track record of working with artificial intelligence. One of the company’s earliest and most famous cases was the 1997 chess match between the blessed world champion and Deep Blue software. It was the first time in history that a computer had beaten a pro-level player.
In 2011, Watson, another AI from IBM, launched Jeopardy!, a competition that provides answers about popular culture and where participants have to guess which question to ask. won the TV show. The software beat out the program’s two biggest human competitors with only access to the company’s offline database. wikipedia🇧🇷
After reaching these two milestones in the history of Artificial Intelligence, IBM began offering services in 2014 for the creation of near-human cognitive applications such as speech and text comprehension, among others.
Evolution of Artificial Intelligence
Deep Blue calculated a simple state space search on the board. The number of possible moves in the game is limited, but absurdly large. A team of chess players helped the program eliminate pointless moves. The computer also used sorting by: Point Decide on each situation moves in limited time.
Although revolutionary at the time, most chess programs today are no longer based on this approach but on Machine Learning, which makes it nearly impossible for a human to beat a computer.
After all, what is the concept of “expert systems” referring to artificial intelligence?
The concept of Artificial Intelligence covers three types of systems in general. The first, known as “expert systems”, It is concerned with the simulation of human intelligence in direct responses to certain actions in the environment.Like Deep Blue or even a simple water flow control system when filling a tank.
These systems manage to reduce the computational complexity of problems through well-defined general rules and depend on the help of a human expert. This strategy dominated the field of AI in the 1970s and 1980s, but it has limited capacity and cannot be used with more complex rules.
However, this does not mean that other technologies such as algorithms were absent, but such a tool lacked processing and storage capacity, as well as a minimal amount of data that was not available at the time.
Big Data Explosion Possible Following the Rise of Artificial Intelligence
The reduction in electronics cost has resulted in increased computational power, not to mention reduced equipment size and increased energy efficiency. This revolution started with processing and storage capabilities, powered by the Internet of Things (IoT) and the spread of social networks, creating the Big Data explosion.
For example, in 1975 the Cray-1 needed 200 kW and was capable of processing 80 megaflops, short for floating point operations per second. Today, the processor has 10,000 times more processing capacity and consumes a thousand times less energy.
Moving a hard drive in 1956 required several people and cost $80,000, but could only hold 5MB of space. Currently, a 1TB SD card is smaller than a fingernail size, as light as a piece of paper, and can be purchased for a modest R$60.
The advent of the internet led to the digitization of the economy. The process is still ongoing and the number of users is increasing every year. Nearly 1 billion people, representing about 17% of the world’s population, used the internet in 2005. In 2019, more than half of the world’s population started using the internet, which is a universe of about 5 billion people.
The information formed as a result of the interaction of this large number of users in applications and social networks on various devices such as smartphones has created a huge database called Big Data. This data is used by organizations to create marketing strategies and can also be useful in developing artificial intelligence.
Does Machine Learning also help the digitization process?
Artificial intelligence has evolved from the possibility of accessing large amounts of data and improving processing and storage to the concept of Machine Learning (ML). Thanks to the algorithm, This technology has the ability to learn from its own mistakes and predict data. without constant interference from humans as in expert systems.
This tool is used in various computational tasks where creating and programming explicit algorithms is impractical. Association rules used in various fields such as ML, Finance and Climatology are applied in approaches such as decision trees and genetic algorithms. The technology is capable of recognizing objects and detecting fraudulent transactions, for example.
And where does deep Learning come from in this story?
There is also a subset of techniques within Machine Learning that can model high-level data abstractions using deep programming with multiple processing layers. Deep Learning (DP) is based on a set of algorithms consisting of various linear and nonlinear transformations.
DP applications are the same as ML applications, the difference between them is in the learning mode. While Machine Learning requires good configuration engineering to accurately describe the features to be analyzed, Deep Learning can do this automatically. In this way, DP is a system completely independent of human intervention, but depends on the quality and quantity of input data.
Did you like the content? Enjoy and stay up to date with other AI-related updates, even in everyday apps like Lensa, which is already a phenomenon on social networks.
Source: Tec Mundo
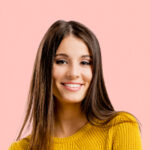