Department of Computer Science graduate student Saloni Kwatra identified the shortcomings of this technology in her thesis and proposed new algorithms to improve user security. He noted that despite the use of federated learning, sensitive information can still be leaked during system updates.
To solve this problem, Saloni used two techniques: k-anonymity and differential privacy. K-anonymity allows data to be organized so that each combination of identifying characteristics (such as height, age, or eye color) belongs to more than one person, making it difficult to identify those individuals individually. Differential privacy ensures that the results of the analysis do not change significantly depending on whether a particular person is included in the data set or not.
Additionally, the researcher also investigated how to protect synthetic data from feature extraction attacks that could threaten privacy. These new algorithms are particularly relevant in areas such as healthcare, finance and telecommunications, where maintaining data integrity is critical. Saloni Kwatra emphasized that the proposed algorithms will help ensure the security of user information and increase the efficiency of the systems.
Source: Ferra
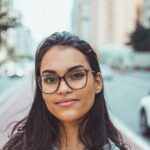
I am a professional journalist and content creator with extensive experience writing for news websites. I currently work as an author at Gadget Onus, where I specialize in covering hot news topics. My written pieces have been published on some of the biggest media outlets around the world, including The Guardian and BBC News.