This discovery may accelerate the development of new alloys and substances in green energy, which is critical to investigate the desired features.
It was revealed that graphic nervous networks trained in low symmetry structures gave almost half of the estimates compared to trained models in high symmetrical samples. At the same time, the volume of training sampling has not changed.
As part of the study, approximately 6 million perovskitic structure with the replacement of various elements was simulated. 1162 representative structure was selected and calculated by using labor intensive quantum-chemical methods. More AI was taught in these data.
Although high symmetrical structures were rare (less than 1% of the entire area), they showed a high feature distribution that could be useful with uncertainty conditions or with non -standard tasks.
The technique already helps to improve hybrid approaches where classical physics are combined with AI. According to the authors, symmetry is not only a feature of the material, but also the key to how machine learning “understands ği the behavior of matter.
Source: Ferra
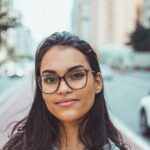
I am a professional journalist and content creator with extensive experience writing for news websites. I currently work as an author at Gadget Onus, where I specialize in covering hot news topics. My written pieces have been published on some of the biggest media outlets around the world, including The Guardian and BBC News.